|
 |
Àâòîðèçàöèÿ |
|
 |
Ïîèñê ïî óêàçàòåëÿì |
|
 |
|
 |
|
 |
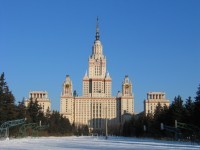 |
|
 |
|
Scott A. — Neuroscience: a mathematical primer |
|
 |
Ïðåäìåòíûé óêàçàòåëü |
McCulloch, Warren 9 11 233 235 237 252
McLeod, J.B. 135
McMullen, T. 279
Meaningful information 9 310
Mel, Bartlett 213 215 217 220 243
Membrane(s) 3 49—65
Membrane(s) batteries 37 38 60—63 73 80 127
Membrane(s) capacitance 31 53—55
Membrane(s) energetics 49 50—52
Membrane(s) model 60—62
Membrane(s) patches 10 219
Membrane(s) permeability 4 37 61—63
Membrane(s) permeability in H-H model 70—73
Membrane(s) pores (channels) 61 76—77
Membrane(s) proteins, intrinsic 28 45 61
Membrane(s) time constant 190 191 199
Memory 8 279—280
Memory in associative neural nets 247—248
Memory in associative neural nets cell assembly theory 261—262 279—280 307—308
Microprobe arrays see Multiple electrode recordings
Milner, Peter 270 272
Mobility, ionic 56
Molecular Dynamics 28—30 34
Molecular dynamics, structure 50
Molecular dynamics, vibrations 33
Mollusk (Aplysia) 284
Momentum density 317
Monkeys, experiments on 284
Moore, John W. 140 141 147 167
Mornev, Oleg 202
Morphogenesis 49
Moth (Manduca sexto) 285
Moving coordinate system 134 323—324
Mueller, Paul 52
Multiple electrode recordings 18 19 285 287
Multiplex neuron 43 44 46 222
Myelinated nerve(s) 6 7 8 27 74 139—159
Myelinated nerve(s) continuum limit 145—146 147 159
Myelinated nerve(s) energy expended in 7 139 140
Myelinated nerve(s) evolutionary design temperature (EDT) 155
Myelinated nerve(s) evolutionary design temperature perspective 153—158 159
Myelinated nerve(s) failure 146 159
Myelinated nerve(s) impulse speed 144—146
Myelinated nerve(s) integrate and fire model 154 156
Myelinated nerve(s) internode conduction time 150
Myelinated nerve(s) internode conduction time predicted for artic fish 158
Myelinated nerve(s) internode conduction time vertebrates 152
Myelinated nerve(s) internode conduction time, general expression for 154
Myelinated nerve(s) internode distance 151 152 157
Myelinated nerve(s) numerical studies 147—148
Myelinated nerve(s) optimal design of 149 153—159
Myelinated nerve(s) saltatory limit 146—147 159
Myelinated nerve(s) statistical properties of 149 150 157—158
Myelinated nerve(s) various vertebrates 152
Myelinated nerve(s) warm- vs. cold-blooded animals 153 155 157 158 159
Myelinated nerve(s), electrical model of 140—144
Na/K—ATPase 64
Nagumo, Jin-ichi 5 123 136
Narcotization of nerve 85—86 126
Navier—Stokes equations 295
Necker cube 262 272—273 280
Negative feedback 13—14 68 304
Neocortical structure 265 307—308
Nernst potential see Diffusion potential
Nernst, Walther 3 59
Nerve cell see Neuron
Nerve impulse(s) 27 31—33 34
Nerve impulse(s), FitzHugh — Nagumo model of 130—132
Nerve impulse(s), Hodgkin—Huxley model of 9 82—84
Nerve impulse(s), leading-edge model of 95—106
Nerve impulse(s), Markin — Chizmadzhev model of 115—122
Nerve impulse(s)on myelinated nerves 7 139—159
Nerve impulse(s)on myelinated nerves squid giant axon 4 84 212
Nets with circles 11 12 18 233 234 241—248
Nets without circles 11 12 233 234—241
Neural models 8—11 19 41—43 187—225
Neural models general structure 25—28
Neural network theory 9 43 233—252
Neuristor(s) 5 104 123 140 199—200
Neuron, generic 25—28 45
Neurotransmitters 35 36 37 44
Newton, Isaac 29 50 294
Newtonian dynamics 28—31 45 58 129 293 295
Newtonian dynamics vs. nonlinear diffusion 33—36
Newtonian dynamics, nature of time in 34 301
Nicolelis, M.A. 282 285
Nicolis, J.S. 309
Noble, D. 199
Nonlinear diffusion 2 5 34 199—206
Nonlinear diffusion discrete 144
Nonlinear diffusion in cortical field theories 248—250
Nonlinear diffusion, equation for 31—33 78 318 324
Nonlinear dynamic hierarchies 28 45 293
Nonlinear dynamic hierarchies biological 293—294
Nonlinear dynamic hierarchies cognitive 305—306
Nonlinear dynamic hierarchies neural 41
Nonlinearity, definition of 300—301
Nonlocal phenomenon 119
Null space 322 332
Numerical models of neurons 220—221
Numerical models of neurons compartmental codes 215 221 222—223
Numerical models of neurons Fourier and Laplace transforms 221
Numerical models of neurons Green functions 221
Numerical models of neurons statistical models 18 222 225
Numerical models of neurons statistically equivalent neurons 220—221
Occipital (optic) lobe 11 307
Offner, F. 103
Ohm’s Law 39 40 77 141
Open systems 34 316
Open systems vs. closed systems 303
Owl, auditory map of 217
Paintel, A.S. 153
Palm, Gunther 276 278
Panizza, Bartolomeo 11
Parietal lobes 307
Parnas, I. 219
Pastushenko, V.F. 105 202
Patch clamp 76
Patterns in context 12
Perceptron 12 233 237—241
Perceptron augmented pattern vector 239
Perceptron learning algorithm 233 237
Perceptron linear discriminant plane 238
Perceptron training period 233
Perceptron training period theorem 240
Perceptron weight vector 238 300
Periaxonal space 87 219
Period of latent addition 235
Perpetual isolation experiments 264—265
Perspiration 13
Perturbation theory for nerve impulses 331—333
Phase plane analysis 99—102
Phase sequence 17 260 264 286 305 308
Phase space 30 32—33 302 303 307
Phase space analysis of F-N equation 5 124—127
Phase space analysis of H-H system 80—82
Phase space equations, autonomous 81
Phase space singular points in 81
Phase space trajectories, heteroclinic vs. homoclinic 81—82 91
Phase space traveling-wave analysis 80—82 91
Phase waves 251
Physicalism 295 306
Piecewise FitzHugh — Nagumo model 123 126
Piecewise linear model 103—104 105
Pitts, Walter 9 11 233 235 237 252
Place cells 287
Planck, Max 59
Planetary motion 30—31 33 129 295
Poggio, T. 221
Poirazi, P. 243
| Polling error 40
Positive feedback 14—17 302—303 304 311
Positive feedback in biological hierarchy 294
Positive feedback in biological hierarchy brain models 234 244
Positive feedback in biological hierarchy cell assemblies 268
Positive feedback in biological hierarchy morphogenesis 252
Positive feedback in biological hierarchy nerve impulse 2 7 79
Postsynaptic membrane 36 37 38
Potassium “turn-on” variable 73 76 95—96 105 319
Potassium “turn-on” variable conductivity 36 62 70 72—73 75
Potassium “turn-on” variable current, components of 62 70 71
Potassium “turn-on” variable ion concentration (in periaxonal space) 87 219
potential energy 29 30 34
Power balance 80 127—129 136 194
Power, definition of 318
Precursor (“skirt”) 119 122
Prediction 30 32
Presynaptic membrane 36 37
Pritchard, R.M. 263
Propagation speed on squid nerve 33 83 84 97—98
Protein Data Bank 30
Proteins 29 300
Proteins immense numbers of 297
Proteins intrinsic 10 53 57 61 76 153
Protobiological molecules 14 15
Psychological time 310
Purkinje cell 207 208
Pyramidal cells 189 215 217 278
Quantum theory 31 34 58
Quick, D.C. 219
Rabbit sciatic nerve 6 7 139 152
Rail, Wilfred 196 205 221
Raminsky, M. 158
Ramon y Cajal, Santiago 8 11 12 208 224
Ramon, F. 167
Ranvier, nodes of see Active nodes
Rapid eye movement (REM) sleep in rats 287
rat 264 285—287
Rayleigh, Lord (John William Strutt) 50
Reaction diffusion equation see Nonlinear diffusion
Reaction time 3 154
Reciprocity theorem 198
Recovery 105 249
Recovery models 115—124
Recovery variable 5 123—124 143
Reductionism, cognitive 306
Reductive materialism 293 294—296
Reductive materialism arguments against 296—305
Reentry 14
Refractory zones 3 5 87—89 91 127
Relative dielectric constant 55
Resting conductance 188
Resting conductance potential 31 63—64 70
Retinal light intensity 13
Retrodiction 30 32
Richer, Ira 146
Rinzel, John 126 221
Rochester, N. 272
Rosenblatt, Frank 12 233 237
Royal Institution of London 2—3
Rudin, Donald 52
Rushton, W.A.H. 153 159
Sabah, N.H. 91
Safety factor for nerve impulse 33 87 111 204
Safety factor in cell assemblies 267 268
Safety factor in cell assemblies Markin—Chizmadzhev model 122
Safety factor in cell assemblies multiplex neuron 10 210
Saltatory conduction 7 139 140 158
Sattinger, D.H. 328
Schmitt, F.O. 44 45
Schmitt, O.H. 166 169
Schrodinger, Erwin 31 35
Schrodinger’s equation 295
Schuster, Peter 309
Sciatic nerve(s) 139—159
Sciatic nerve(s) of cat 149 150 157
Sciatic nerve(s) of frog 1 2 74 142—143 149 150
Sciatic nerve(s) of rabbit 6 7 139
Sciatic nerve(s) ofother vertebrates 152 157 158
Sears, T.A. 158
Senden, Marius von 258 262
Separation of variables 134 325
Sherrington, Charles Scott 8 11 257 311
Shooting method 82 101—102 111
Shot noise 40
Sigma-Pi model of dendrites 214—215
Sigmoid umction 214 245—246 249 267
Silberstein, P.T. 90
Single neuron recording 257
Skou, Jens Christian 64
Smith, B.H. 44 45
Smith, Dean 218 219
Sneyd, James 199
Soap bubble 49 50—51
Soap bubble film 50—51 65
Soap bubble film black 51
Social assemblies 17 261 274
Social assemblies time 310
Sodium channels conductance 36 61—62 64 72—75
Sodium channels current 61—62 70 71
Sodium channels “turn-on” and “turn-off” 72 76 95—96 105 110 154 319—320
Sodium channels, genetic variations of 153
Sodium-potassium pump 63—64 65
solder 243—244
Soliton(s) 80 248
Southampton — Duke Morphological Archive 188 189 220
Soviet Union 5 10 207
Space clamp(ed) 67 68 69 71 91 249 269
Space clamp(ed) squid membrane 71—72 74—77
Space constant 190 191
Space constant active 224
Speed of impulse propagation, myelinated nerve 1 2 7 142 145—147 149 158
Speed of impulse propagation, smooth nerve 3 83 84 97—98 121
Spike response model 42 116
Spin-glass brain model 234 248 252
Spira, M.E. 219
Spreading resistance 40
Spruston, Nelson 45
Squid giant axon 3 4 6—7 27 32 40
Squid giant axon branching GR for 218
Squid giant axon H-H model of 9 77—78 83—84 120
Squid giant axon membrane currents 69 70—74
Squid giant axon membrane currents oscillatory behavior of 90—91
Squid giant axon membrane currents permeabilities 63 70—74
Squid giant axon nerve impulse, stability 83—84 86
Squid giant axon, impulse speed for 83 97—98
Squid giant axon, refractory zones of 87—89
Stability 14 323—330
Stability Lyapunov 246
Stability of attractor neural network 245
Stability of attractor neural network M-C impulses 121
Stability of attractor neural network, axonal impulses 323—330
Stability of attractor neural network, cell assemblies 268 272 273—274
Stability of attractor neural network, F-N impulses 132—136
Stability of attractor neural network, H-H impulse 83 84
Stability of attractor neural network, leading edges 108—109
Stampfli, R. 158
State diagrams 241—242
Statistical models of neural nets 18
Statistical models of neurons 222
Stimulus-response problems 321
Stochastic behavior of synapses 38
Stuart, Greg 45
Studies of Artificial Neural Systems (SANS) 278 284
Subjective experience 311
Subthreshold resonance 91
Superposition theorem 193
Supervenience 295 296
Synapses 8 10 27 248
Synapses active and passive 215—216
|
|
 |
Ðåêëàìà |
 |
|
|