|
 |
Àâòîðèçàöèÿ |
|
 |
Ïîèñê ïî óêàçàòåëÿì |
|
 |
|
 |
|
 |
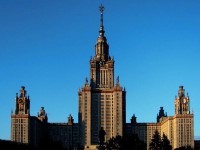 |
|
 |
|
Verbeke G., Molenberghs G. — Linear Mixed Models for Longitudinal Data |
|
 |
Ïðåäìåòíûé óêàçàòåëü |
Age-related macular degeneration 427
Age-related macular degeneration, coefficient of multiple determination 437
Akaike information criterion (AIC) see “Information criteria”
Ancillary statistic 377
Ancova 221
anova 221 229
Autoregressive AR(1) see “Covariance structure”
Available case analysis 222 227 263
Balanced data 119 215
BALANCED object see “OSWALD”
Baltimore Longitudinal Study of Aging (BLSA) 10—12
Baltimore Longitudinal Study of Aging (BLSA), hearing data see “Hearing data”
Baltimore Longitudinal Study of Aging (BLSA), prostate data see “Prostate data”
Banded see “Covariance structure”
Bayesian methods 41 46 69 470
Bayesian methods, empirical Bayes estimation (EB) see “Random effects”
Bayesian methods, hierarchical Bayes model 172
Bayesian methods, posterior distribution 78 176
Bayesian methods, posterior mean 78 176
Bayesian methods, posterior probability 175 177
Bayesian methods, prior distribution 78
Bayesian methods, shrinkage 80—82 85
Best linear unbiased prediction (BLUP) 80
Bivariate outcome 405
Blood pressure data 405—411
Bozdogan information criterion (CAIC) see “Information criteria”
BY statement 357
Case deletion see “Global influence”
Class statement 96 483
Classification of profiles see “Heterogeneity model”
Cluster analysis see “Heterogeneity model”
Coefficient of multiple determination, random effect 431 438
Coefficient of multiple determination, residual 433 438
Coefficient of multiple determination, subject-specific see “Subject-specific profiles”
Colorectal cancer 427—129
Colorectal cancer, coefficient of multiple determination 438
Complete case analysis 211 222—223 227
Complete data see “Missing data”
Complete data set 241
Compound symmetry 25 68 117 118
Compound symmetry, Greenhouse — Geiser 120
Compound symmetry, Huynh — Feldt 120
Compound symmetry, random intercepts see “Random-intercepts model”
Computing time 47 97 118
Conditional independence see “Covariance structure”
Conditional linear mixed model 194—197
Conditional linear mixed model, empirical Bayes estimation (EB) 195
Conditional linear mixed model, estimation 195
Conditional linear mixed model, fixed-effects approach 198—200
Conditional linear mixed model, inference 195
Conditional linear mixed model, justification 196
Conditional linear mixed model, maximum likelihood estimation (ML) 195
Conditional linear mixed model, paired t-test 196
Conditional linear mixed model, restricted maximum likelihood estimation (REML) 195
CONTRAST statement 101 104 410
CONTRAST statement, “chisq” option 101
Convergence problems see “Estimation problems”
Cook's distance see “Influence analysis”
Covariance structure 62 64 117 121 240 265
Covariance structure, ante-dependence 232
Covariance structure, autoregressive AR(1) 99 122 252 258 388 452 499
Covariance structure, banded 99 388
Covariance structure, compound symmetry 25 68 99 117 118 120 253 260 262 302—307 326 389
Covariance structure, conditional independence 26 85 159
Covariance structure, correlation structure 34 419
Covariance structure, exchangeable 253 260 388
Covariance structure, exploration 33 34
Covariance structure, heterogeneous 99
Covariance structure, independence 106 256 389
Covariance structure, measurement error 27 28 117 129 135 237 241 270 416 502 504
Covariance structure, model building 125 132
Covariance structure, patterned 388
Covariance structure, preliminary 474
Covariance structure, random effects 28 135 416
Covariance structure, residual 26—29 128 132 271 416
Covariance structure, residual, REPEATED statement 98
Covariance structure, residuals 160
Covariance structure, serial correlation 27 28 128—132 135—150 241 306 416 449 498
Covariance structure, serial correlation, exponential 28 100 139 142
Covariance structure, serial correlation, Gaussian 28 100 129 139 142 270
Covariance structure, simple 99
Covariance structure, spatial 100
Covariance structure, starting values 131
Covariance structure, stationarity 126 127 142
Covariance structure, Toeplitz 99 250 262
Covariance structure, unstructured 99 242 247 353 389
Covariance structure, variance function 25 33 53 127 130 131 201
Coverage probability 382
Cross-sectional component 189 194
Degrees of freedom, F-test see “F-test”
Degrees of freedom, Satterthwaite method 57 112 486
Degrees of freedom, t-test see “t-test”
Delta method 286 357 432 451 454
design considerations 391 104
Design considerations, comparing power distributions 395 397—404
Design considerations, designed power 391 394—397
Design considerations, dropout mechanism 397
Design considerations, intentionally incomplete 393
Design considerations, power 392—393
Design considerations, power distribution 394—404
Design considerations, random-effects distribution 87
Design considerations, realized power 391 394 104
Design considerations, sampling methods 396 104
Design considerations, under expected dropout 394—397
Design matrix 215 241 247 249 252 259
Deviance 242
Discriminant analysis see “Heterogeneity model”
Dose-response model 412
Dropout see “Missing data”
Dropout model 235 236 269 271 280 297 302 314 326 328
Dropout model, direct variables 465—466 469
Dropout model, increment 272 312 315 321 326 328 463 465—466 469
Dropout model, size 272 312 315 326
Dropout rate 280
Efficiency see “Design considerations”
EM algorithm 173 177 329 387—390
EM algorithm, E step 175 388
EM algorithm, ECM algorithm 387
EM algorithm, ECME algorithm 387
EM algorithm, GEM algorithm 389
EM algorithm, heterogeneity model 173—177
EM algorithm, linear mixed model 47
EM algorithm, M step 175 388
EM algorithm, missing data 210 222 232 240 258 277 302 376
EM algorithm, rate of convergence 47 389
EM algorithm, versus Newton — Raphson 173
Empirical Bayes estimation (EB) see “Random effects”
Empirical variance see “Fixed effects”
Error contrasts see “REML”
ESTIMATE statement 101 104 410
ESTIMATE statement, “alpha=” option 102
ESTIMATE statement, “cl” option 102
Estimation problems 50—54 439—442
Estimation problems, model misspecification 52—54
Estimation problems, model parameterization 131
Estimation problems, small variance components 50—52
Exchangeable see “Covariance structure”
Exercise bike data 498
Exponential serial correlation see “Serial correlation”
F-test, degrees of freedom 57 112 393
F-test, fixed effects see “Fixed effects”
F-test, noncentral 393 396
F-test, PROC MIXED versus PROC GLM 119
F-test, random effects see “Random effects”
Fisher scoring 50 103 131 385
Fixed effects 24 224 241 385
Fixed effects, F-test 56 112 115 392
Fixed effects, general linear hypothesis 56 58 392
Fixed effects, general linear hypothesis, CONTRAST statement 101
Fixed effects, inference 55—63 133
| Fixed effects, local influence see “Local influence”
Fixed effects, LR test 62 392
Fixed effects, maximum likelihood 42
Fixed effects, MODEL statement 96
Fixed effects, multivariate test 119
Fixed effects, parameterization in SAS 114—117
Fixed effects, restricted maximum likelihood 45
Fixed effects, robust inference 61—62 121
Fixed effects, robust inference, empirical variance 61 474
Fixed effects, robust inference, sandwich estimator 61 88
Fixed effects, t-test 56 111 112 115 392
Fixed effects, versus random effects 198—200
Fixed effects, Wald test 56 112
Fractional polynomials 20 137—139 478—479
Full data see “Missing data”
Gaussian serial correlation see “Serial correlation”
General linear hypothesis see “Fixed effects”
Generalized estimating equations 125 218 229 391
Gibbs sampling 390
Global influence 314 460 162
Global influence, case deletion 153 165 298 314 320 325 457
Global influence, one-step approach 152 159
Global influence, versus local influence 153 466—470
Greenhouse — Geiser see “Compound symmetry”
Growth curves 248
Growth data 16—17 240—268 388
Growth data, complete data analysis 240—256
Growth data, incomplete data analysis, frequentist analysis 256—257
Growth data, incomplete data analysis, likelihood analysis 257—267
Growth data, incomplete data analysis, missinguess process 267—268
Growth data, MLwiN 489—493
Growth data, multilevel model 489—493
Hannan and Quinn information criterion (HQC) see “Information criteria”
Hearing data 14
Hearing data, conditional linear mixed model 197—200
Hearing data, contaminated data 191 193
Hearing data, empirical Bayes estimation (EB) 192
Hearing data, fixed effects versus random effects 198—200
Hearing data, linear mixed model 190 191
Hearing data, misspecified cross-sectional component 191—193
Heat shock study 411—419
Heights of schoolgirls 16
Heights of schoolgirls, classification of subjects 184—187
Heights of schoolgirls, cluster analysis 184—187
Heights of schoolgirls, discriminant analysis 184—187
Heights of schoolgirls, heterogeneity model 184—187
Heights of schoolgirls, linear mixed model 183—184
Heights of schoolgirls, two-stage approach 183
Henderson's mixed model equations 79
Hepatitis B vaccination 470 484
Hepatitis B vaccination, semi-variogram 473
Hessian matrix see “Information matrix”
Heterogeneity model 85 87 90 91 169 171—172
Heterogeneity model, classification 169 177 182 186
Heterogeneity model, cluster analysis 177 181 186
Heterogeneity model, discriminant analysis 177 181
Heterogeneity model, EM algorithm 173—177
Heterogeneity model, empirical Bayes estimation (EB) 176
Heterogeneity model, goodness-of-fit 178 179 181 185
Heterogeneity model, identifiability 174
Heterogeneity model, Kolmogorov — Smirnov test 178 181 186
Heterogeneity model, likelihood ratio test 91 178
Heterogeneity model, Newton — Raphson 173
Heterogeneity model, number of components 91 178—179 181 185
Heterogeneity model, posterior probability 175 177
Heterogeneity model, Shapiro — Wilk test 179 181 186
Hierarchical Bayes model see “Bayesian methods”
Hierarchical model 24 41 52 65 67 69 77 117
Hierarchical model, versus marginal model 52 65 117
Homogeneity model 85 90 172
Homogeneity model, goodness-of-fit 178—179 181 185
Hot deck imputation see “Imputation”
Huynh — Feldt see “Compound symmetry”
ID statement 97
Identifiability 174 231 278 280
Identifiable parameter 216 (see also “Estimable parameter”)
Identifying restrictions see “Pattern-mixture model”
Ignorable analysis 239—240 266
Ignorable missing data see “Missing data”
Imputation 222
Imputation, Buck 225
Imputation, conditional mean 224 226
Imputation, hot deck 224 226
Imputation, last observation carried forward (LOCF) 224 226
Imputation, mean 224
Imputation, multiple 222 336 339 344 350—352 359 371 373
Imputation, multiple, estimation 338
Imputation, multiple, estimation task 337
Imputation, multiple, F-test 339
Imputation, multiple, hypothesis testing 338—339
Imputation, multiple, imputation task 337
Imputation, multiple, modeling task 337
Imputation, multiple, proper 344
Imputation, multiple, variance 338
Imputation, simple 211—212 222—226
Imputation, single 223
Imputation, unconditional mean 225
Incomplete data see “Missing data”
Independence model see “Covariance structure”
Influence analysis 457—470
Influence analysis, Cook's distance 151 457
Influence analysis, global 305 (see “Global influence hat-matrix diagonal”)
Influence analysis, leverage 151 304
Influence analysis, local see “Local influence”
Influence graph see “Local influence”
Information criteria 74—76 129 409
Information criteria, Akaike (AIC) 74 106 107 406
Information criteria, Bozdogan (CAIC) 74 107
Information criteria, Hannan and Quinn (HQIC) 74 107
Information criteria, ML versus REML 75 107
Information criteria, Schwarz (SBC) 74 106 107 406
Information matrix 64 88
Information matrix, expected 103 131 132 376 378 382
Information matrix, Hessian 50 131
Information matrix, naive 380
Information matrix, observed 103 131 132 376 378 385
Intentionally incomplete designs 393
Intraclass correlation 25 68 86 260
Kolmogorov — Smirnov test 178 181 186
kurtosis 318
Last observation carried forward see “Imputation”
Latent variable 328
Leverage see “Influence analysis”
Likelihood displacement see “Local influence”
Likelihood function 104 106
Likelihood function, complete data 378
Likelihood function, factorization 217
Likelihood function, full data 217
Likelihood function, maximum likelihood 42
Likelihood function, objective function 105
Likelihood function, observed data 258 318 387
Likelihood function, restricted maximum likelihood 46
Likelihood ratio test 239 375
Likelihood ratio test, asymptotic null distribution 69—73 91 178 254
Likelihood ratio test, fixed effects 62 247 392
Likelihood ratio test, heterogeneity model 91 178
Likelihood ratio test, missing data 375
Likelihood ratio test, missing data mechanism 310 313 320 324 459 511
Likelihood ratio test, ML versus REML 63 66 69
Likelihood ratio test, pattern specific 283
Likelihood ratio test, random effects 69—73 133 408
Likelihood ratio test, variance components 65 106 133 262 392
Likelihood ratio test, Wilk's Lambda 119
Likelihood-based frequentist inference 385
Linear mixed model 23—29
Local influence 153 158 298—300
Local influence, case-weight perturbation 156
Local influence, cutoff value 161 162
Local influence, fixed effects 161 162 303
Local influence, index plot 160 161 163
|
|
 |
Ðåêëàìà |
 |
|
|