|
 |
Àâòîðèçàöèÿ |
|
 |
Ïîèñê ïî óêàçàòåëÿì |
|
 |
|
 |
|
 |
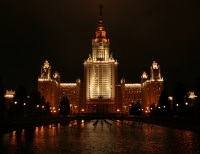 |
|
 |
|
Rencher A.C. — Methods of multivariate analysis |
|
 |
Ïðåäìåòíûé óêàçàòåëü |
Growth curves, one sample 221—229
Growth curves, orthogonal polynomials 222—225
Growth curves, polynomial function of t 225—227
Growth curves, several samples 229—230
Growth curves, unequally spaced time points 225—227
Guinea pig data 201
H matrix 160—161 343—344
Height-weight data 45
Hematology data 109—110
Hierarchical clustering see Cluster analysis hierarchical
Hotelling — Lawley test statistic see Lawley — Hotelling test statistic
Hotelling's -statistic see -statistic
Hyperellipsoid 73
Hypothesis tests see Tests of hypotheses
Identity matrix 8
Imputation 74
Independence of variables, test for 265—266
Independence of variables, test for, table of exact critical values 590
Indicator variables see Dummy variables
Inferential statistics 2
Intra-class correlation 198—199
Kernel density estimators 315—317
kurtosis 94—95 98—99 103—104
Largest root test see Roy's test statistic
Latent roots see Eigenvalues
Lawley — Hotelling test statistic: definition of 167
Lawley — Hotelling test statistic: table of critical values 582—586
Length of vector 14
Likelihood function 90
Likelihood ratio test(s): for covariance matrices 248—250 253 256 260 262 265
Likelihood ratio test(s): for mean vectors 126 164
Likelihood ratio test(s): in factor analysis 428
Linear classification functions 301—306
Linear combination of matrices 19
Linear combination of vectors 19
Linear combination(s) of variables 2 67—73 113
Linear combination(s) of variables, correlation matrix for several linear combinations 72
Linear combination(s) of variables, correlation of two linear combinations 67 71—73
Linear combination(s) of variables, covariance matrix for several linear combinations 69—70 72—73
Linear combination(s) of variables, covariance of two linear combinations 67—68 71—72
Linear combination(s) of variables, distribution of 86
Linear combination(s) of variables, mean of a single linear combination 67 71—72
Linear combination(s) of variables, mean vector for several linear combinations 69
Linear combination(s) of variables, variance of a single linear combination 67 71—72
Linear hypotheses 141—142 199—201 208—225
Mahalanobis distance 76—77 83
Mandible data 247
Manova 130 158. multivariate
Matrix (matrices): algebra of 5—37
Matrix (matrices): bilinear form 19—20
Matrix (matrices): Burt matrix 526—529
Matrix (matrices): Cholesky decomposition 25—26
Matrix (matrices): conformable 11
Matrix (matrices): covariance matrix 57—59
Matrix (matrices): definition 5—6
Matrix (matrices): determinant 26—29 34.
Matrix (matrices): determinant of diagonal matrix 27
Matrix (matrices): determinant of inverse matrix 29
Matrix (matrices): determinant of partitioned matrix 29
Matrix (matrices): determinant of positive definite matrix 28
Matrix (matrices): determinant of product 28
Matrix (matrices): determinant of scalar multiple of a matrix 28
Matrix (matrices): determinant of singular matrix 28
Matrix (matrices): determinant of transpose 29
Matrix (matrices): diagonal 8
Matrix (matrices): eigenvalues 32—37. See also Eigenvalues
Matrix (matrices): eigenvalues and determinant 34
Matrix (matrices): eigenvalues and trace 34
Matrix (matrices): eigenvalues of I+A 33
Matrix (matrices): eigenvalues of inverse matrix 36
Matrix (matrices): eigenvalues of positive definite matrix 34
Matrix (matrices): eigenvalues of positive definite matrix, Perron — Frobenius theorem 34
Matrix (matrices): eigenvalues of positive definite matrix, square root matrix 36
Matrix (matrices): eigenvalues of product 35
Matrix (matrices): eigenvalues of square matrix 36
Matrix (matrices): eigenvalues of symmetric matrix 35
Matrix (matrices): eigenvalues of symmetric matrix, spectral decomposition 35
Matrix (matrices): eigenvalues, characteristic equation 32
Matrix (matrices): eigenvalues, singular value decomposition 36
Matrix (matrices): eigenvectors 32—37. See also Eigenvectors
Matrix (matrices): equality of 7
Matrix (matrices): identity 8
Matrix (matrices): indicator matrix 526—527
Matrix (matrices): inverse 23—25
Matrix (matrices): inverse of partitioned matrix 25
Matrix (matrices): inverse of product 24
Matrix (matrices): inverse of transpose 24
Matrix (matrices): J matrix 9
Matrix (matrices): j vector 9
Matrix (matrices): linear combination of 19
Matrix (matrices): nonsingular matrix 23
Matrix (matrices): notation for matrix and vector 5—6
Matrix (matrices): O (zero matrix) 9
Matrix (matrices): operations with 9—20
Matrix (matrices): operations with distributive law 12
Matrix (matrices): operations with factoring 12—13 15
Matrix (matrices): operations with product 11—20 23—25
Matrix (matrices): operations with product and eigenvalues 34—35
Matrix (matrices): operations with product of matrix and scalar 19
Matrix (matrices): operations with product of matrix and transpose 16—18
Matrix (matrices): operations with product of matrix and vector 12—13 16 21
Matrix (matrices): operations with product of matrix and vector as linear combination 21
Matrix (matrices): operations with product of vectors 14
Matrix (matrices): operations with product, conformable 11
Matrix (matrices): operations with product, distributive over addition 12
Matrix (matrices): operations with product, noncommutativity of 11
Matrix (matrices): operations with product, product equal zero 23
Matrix (matrices): operations with product, transpose of 12
Matrix (matrices): operations with product, triple product 13
Matrix (matrices): operations with product, with diagonal matrix 18
Matrix (matrices): operations with sum 10
Matrix (matrices): operations with sum, commutativity of 10
Matrix (matrices): orthogonal 31
Matrix (matrices): orthogonal, rotation of axes 31—32
Matrix (matrices): partitioned matrices 20—22
Matrix (matrices): partitioned matrices, determinant of 29
Matrix (matrices): partitioned matrices, inverse of 25
Matrix (matrices): partitioned matrices, product of 20—21
Matrix (matrices): partitioned matrices, transpose of 22
Matrix (matrices): Perron — Frobenius theorem 34 402
Matrix (matrices): positive definite 25 34
Matrix (matrices): positive semidefinite 25 34
Matrix (matrices): quadratic form 19
Matrix (matrices): rank 22—23
Matrix (matrices): rank, full rank 22
Matrix (matrices): scalar 6
Matrix (matrices): scalar, product of scalar and matrix 19
Matrix (matrices): singular matrix 24
Matrix (matrices): singular value decomposition 36
Matrix (matrices): size of a matrix 6
Matrix (matrices): spectral decomposition 35
Matrix (matrices): square root matrix 36
Matrix (matrices): sum of products in vector notation 14
Matrix (matrices): sum of squares in vector notation 14
Matrix (matrices): symmetric 7 35
Matrix (matrices): trace 30 34 69
Matrix (matrices): trace and eigenvalues 34
Matrix (matrices): trace of product 30
Matrix (matrices): trace of sum 30
Matrix (matrices): transpose 6—7
Matrix (matrices): transpose of product 12
Matrix (matrices): transpose of sum 10
Matrix (matrices): triangular 8
Matrix (matrices): vectors see Vector(s)
Matrix (matrices): zero matrix (O) and zero vector (0) 9
Maximum Likelihood Estimation 90—91
Maximum likelihood estimation of correlation matrix 91
Maximum likelihood estimation of covariance matrix 90
Maximum likelihood estimation of mean vector 90—91
Maximum likelihood estimation, likelihood function 90
| Maximum likelihood estimation, multivariate normal 90
Mean vector 54—56 83 90—92
Mean vector, notation 54
Mean vector, population mean vector 55—56
Mean vector, sample mean vector 54—56
Mean vector, sample mean vector and sample covariance matrix, independence of 92
Mean vector, sample mean vector from data matrix 55
Mean vector, sample mean vector , distribution of 91
Mean: geometric 174
Mean: of linear function 67 72
Mean: of product 46
Mean: of sum 46
Mean: population mean 43
Mean: sample mean 43—44
Measurement scale 2
Measurement scale, interval scale 2
Measurement scale, ordinal scale 2
Measurement scale, ratio scale 2
Mice data 241
Misclassification rates see Error rate(s)
Missing values 74—76
Multicollinearity 74 84
Multidimensional scaling 504—514
Multidimensional scaling, classical solution see Metric multidimensional scaling
Multidimensional scaling, definition 504—505
Multidimensional scaling, distances 504—505
Multidimensional scaling, distances, seriation (ranking) 504
Multidimensional scaling, metric multidimensional scaling 504—508
Multidimensional scaling, metric multidimensional scaling and principal component analysis 506
Multidimensional scaling, metric multidimensional scaling, algorithm for finding the points 505—508
Multidimensional scaling, nonmetric multidimensional scaling 505 508—514
Multidimensional scaling, nonmetric multidimensional scaling, monotonic regression 509—510
Multidimensional scaling, nonmetric multidimensional scaling, ranked dissimilarities 508—509
Multidimensional scaling, nonmetric multidimensional scaling, STRESS 510—512
Multidimensional scaling, principal coordinate analysis see Metric multidimensional scaling
Multidimensional scaling, spectral decomposition 505—506
Multiple correlation 332 361—362 423.
Multiple correspondence analysis 526—530
Multiple correspondence analysis, Burt matrix 526—529
Multiple correspondence analysis, column coordinates 527 529—530
Multiple correspondence analysis, indicator matrix 526—527
Multiple regression see Regression multiple
Multivariate analysis 1
Multivariate analysis of variance (Manova) see Analysis of variance multivariate
Multivariate analysis, descriptive statistics 1—2
Multivariate analysis, inferential statistics 2
Multivariate data: basic types of 4
Multivariate data: plotting of 52—53
Multivariate data: sparceness of 97
Multivariate inference 2
Multivariate normal distribution 82—105
Multivariate normal distribution, applicability of 85
Multivariate normal distribution, conditional distribution 88
Multivariate normal distribution, contour plots 84—85
Multivariate normal distribution, density function 83
Multivariate normal distribution, distribution of and S 91—92
Multivariate normal distribution, features of 82
Multivariate normal distribution, independence of and S 92
Multivariate normal distribution, linear combinations of 86
Multivariate normal distribution, marginal distribution 87
Multivariate normal distribution, maximum likelihood estimates 90—91. See also Maximum likelihood estimation
Multivariate normal distribution, properties of 85—90
Multivariate normal distribution, quadratic form and chi-square distribution 86
Multivariate normal distribution, standardized variables 86
Multivariate normal distribution, zero covariance matrix implies independence of subvectors 87
Multivariate normality, dynamic plot 98
Multivariate normality, scatter plots 98 105
Multivariate normality, skewness and kurtosis, multivariate 98—99 103—104 106
Multivariate normality, skewness and kurtosis, table of critical values 553—556
Multivariate normality, tests for 92 96—99
Multivariate normality, tests for 97—98 102—103
Multivariate normality, tests for and chi-square 98
Multivariate normality, tests for table of critical values 557
Multivariate regression see Regression multivariate
Nonsingular matrix 23
Normal distribution: bivariate normal 46 84 88—89 133
Normal distribution: multivariate normal see Multivariate normal distribution
Normal distribution: univariate normal 82—83 86
Normality, tests for see Multivariate normality; Univariate normality
Norway crime data 544
Numerical taxonomy see Cluster analysis
Objectives of this book 3
Observations 1
One-sample test for a mean vector 117—121
Orthogonal matrix 31
Orthogonal polynomials 222—225
Orthogonal polynomials, table of 587
Orthogonal vectors 50
Outliers: multivariate: kurtosis 103—104
Outliers: multivariate: kurtosis, elliptically symmetric distributions 103
Outliers: multivariate: principal components 389—392
Outliers: multivariate: slippage in mean, variance, and correlation 101
Outliers: multivariate: Wilks' statistic 102—103
Outliers: univariate: accommodation 100
Outliers: univariate: block test 101
Outliers: univariate: identification 100
Outliers: univariate: masking 101
Outliers: univariate: maximum studentized residual 100—101
Outliers: univariate: skewness and kurtosis 101
Outliers: univariate: slippage in mean and variance 100
Outliers: univariate: swamping 101
Overall variability 73—74
Paired observation test 132—136
Partial F-tests 127 138 232 293—296
Partitioned matrices see Matrix (matrices) partitioned
Partitioning see Cluster analysis partitioning
Pattern recognition see Cluster analysis
People data 526
Perception data 419
Perron — Frobenius theorem 34 402
Pillai's test statistic: definition of 166
Pillai's test statistic: table of critical values 578—581
Piston ring data 518
Plasma data 246
Plotting multivariate data 52—53
Politics data 542
Positive definite matrix 25
Positive definite matrix, positive definite sample covariance matrix 67
Prerequisites for this book 3
Principal components 380—407
Principal components and biplots 531—532
Principal components and cluster analysis 390—393 395 482—484 487
Principal components and factor analysis 403 408—409 447—448
Principal components and perpendicular regression 385 387—389
Principal components as rotation of axes 381—382 384—385
Principal components from S or R 383—384 393—397
Principal components from S or R, nonuniqueness of components from R 397
Principal components, algebra of 385—387
Principal components, component scores 386
Principal components, definition of 380 382 385
Principal components, dimension reduction 381—384 385—387 389
Principal components, eigenvalues and eigenvectors 382—385 397—398
Principal components, eigenvalues and eigenvectors, major axis 384 388
Principal components, geometry of 381—385
Principal components, interpretation 401—404
Principal components, interpretation, correlations 403—404
Principal components, interpretation, rotation 403
Principal components, interpretation, special patterns in S or R 401—403
Principal components, interpretation, special patterns in S or R, size and shape 402—403
Principal components, large variance of a variable, effect of 383—384 402
Principal components, last few principal components 382 389 401
Principal components, maximum variance 380 385
Principal components, minimum perpendicular distances to line 387—388
Principal components, number of components to retain 397—401
Principal components, orthogonality of 380 383—384
Principal components, percent of variance 383 397
Principal components, plotting of 389—393
Principal components, plotting of assessing normality 389—390
Principal components, plotting of detection of outliers 389—391
Principal components, properties of 381—386
|
|
 |
Ðåêëàìà |
 |
|
|