|
 |
Àâòîðèçàöèÿ |
|
 |
Ïîèñê ïî óêàçàòåëÿì |
|
 |
|
 |
|
 |
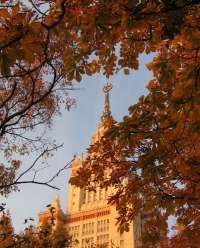 |
|
 |
|
Neter J., Kutner M.H., Wasserman W. — Applied Linear Regression Models |
|
 |
Ïðåäìåòíûé óêàçàòåëü |
"Best" subsets algorithms 429
criterion 426—428
criterion 423—425
criterion 423—425
criterion 422—423
Addition theorem 2
Adjusted coefficient of multiple determination 241—242
All-possible-regressions selection procedure 421—429
Allocated codes 351—352
Analysis of variance 84—86
Analysis of variance models 343
Analysis of variance table 89—90
ANOVA table 89—90
Asymptotic normality 70
Autocorrelation 444—448
Autocorrelation parameter 448
Autocorrelation, remedial measures 454—460
Autocorrelation, test for 450—454
Autoregressive error model see "Regression model"
Backward elimination selection procedure 435—436
Berkson model 166—167
Beta coefficient 262
Biased estimation 394—395
Binary variable 330 354 see
Bivariate normal distribution 492—496
BMDP 113 251 431
Bonferroni joint estimation procedure for inverse predictions 174
Bonferroni joint estimation procedure for mean responses 158—159 245
Bonferroni joint estimation procedure for prediction of new observations 159—160 246—247
Bonferroni joint estimation procedure for regression coefficients 150—154 243
Calibration problem 174
Central limit theorem 6
Chi-square distribution 7—8
Chi-square distribution, table of percentiles 520
Cochran's theorem 92
Coefficient of multiple correlation 242 506
Coefficient of multiple correlation, inferences 506—507
Coefficient of multiple determination 241 506
Coefficient of multiple determination, adjusted 241—242
Coefficient of multiple determination, inferences 506—507
Coefficient of partial correlation 288—289 507—508
Coefficient of partial correlation, first-order 508
Coefficient of partial correlation, inferences 508
Coefficient of partial correlation, second-order 508
Coefficient of partial determination 286—288 507—508
Coefficient of partial determination, inferences 508
Coefficient of simple correlation 97—99 494
Coefficient of simple correlation, inferences 502—504
Coefficient of simple determination 96—97 501—502
Coefficient of simple determination, inferences 502—504
Column vector 187
Complementary event 3
Conditional probability 3
Conditional probability function 4
Confidence coefficient, interpretation of 70—71 84
Confidence set 152
Consistent estimator 9
Contour diagram 235—237 494—495
Cook's distance measure 407—409
Correction for mean sum of squares 90
Correlation coefficient see "Coefficient of multiple correlation" "Coefficient "Coefficient
Correlation Index 312
Correlation matrix 382
Correlation matrix of the independent variables 381
Correlation model 491—492
Correlation model, bivariate normal 492—496
Correlation model, multivariate normal 505
Correlation transformation 378—379
Covariance models 343
Covariance of two functions of random variables 6
Covariance of two random variables 5
Cox, D.R. 176
Degrees of freedom 7—8
Deleted residual 405—406
Denominator degrees of freedom 8
Dependent variable 25 28
Determinant of matrix 202
Diagonal matrix 196—197
Disturbance term 445
Dummy variable 34 330 see
Durbin — Watson test 450—454
Durbin — Watson test, table of test bounds 530—531
Error mean square 47
Error sum of squares 47
Error term 31
Error term variance 31 46—48 50
Error term, nonconstancy of error variance 113—114 123 133
Error term, nonindependence of 116—118 123 133
Error term, nonnormality of 118—120 123 133
Expected mean square 90—91
Expected value of function of random variables 5—6
Expected value of random variable 3
Experimental data 35
Exponential regression function 468
Extra sum of squares 282—286
F distribution 8—9
F distribution, table of percentiles 521—527
Family confidence coefficient 150
Family of estimates 150
First differences 458—459
First-order autoregressive error model 448—450
First-order autoregressive error model, first differences approach 458—460
First-order autoregressive error model, iterative estimation approach 455—458
First-order autoregressive error model, test for autocorrelation 450—454
First-order regression model 31 227 229—230 see
Fisher, R.A. 503
Fitted value 41
Fitted value in terms of hat matrix 401
Forward selection procedure 435
Full model 95
Functional relation 24
Gauss — Markov theorem 39—40 64
Gauss — Newton method 472—479
General linear regression model 230—234 237—238
General linear test 94—96 293—296
Hat matrix 220—221
Heteroscedasticity 170
Homoscedasticity 170
Hyperplane 230
Idempotent matrix 221
Identity matrix 197
Independence of random variables 5
Independent variable 25 28
Indicator variable 329—330 353—354
Indicator variable in comparing regression functions 343—345
Indicator variable in piecewise linear regression 346—350
Indicator variable in time series model 350—351
Indicator variable, as dependent variable 354—357
Influential observations 407—409
Instrumental variable 165—166
Interaction effect 232—237
Interaction effect coefficient 304
Interaction effect with indicator variables 335—339
Intrinsically linear regression model 467
Inverse of matrix 200—204
Inverse prediction 172—174
Joint confidence region for regression coefficients 147—150 217 243
Joint probability function 4
Lack of fit mean square 129
Lack of fit sum of squares 128
Lack of fit test 123—132 245—246
Least absolute deviations estimation 410—411
Least squares criterion 36
Least squares estimation 10
Least squares estimation, control of roundoff errors 377—382
Least squares estimation, multiple regression 238—239
Least squares estimation, nonlinear regression 470—480
Least squares estimation, simple linear regression 36—40 44—46 210—212
Least squares estimation, weighted 167—172 219—220 263
Leverage 402
| Likelihood function 9
Linear dependence 199—200
Linear effect coefficient 301
Linear model 31 466—467 see
Linear model, general linear test 94—96 293—296
Linear regression model see "Regression model"
Linearity, test for 123—132
Logistic regression function 361—362 468—469
Logistic transformation 362
Logit transformation 362
Marginal probability function 4
Marquardt algorithm 479—80
Matrix of quadratic form 215
Matrix with all elements 1 198
Matrix, addition 190—191
Matrix, definition 185—187
Matrix, determinant 202
Matrix, diagonal 196—197
Matrix, dimension 186
Matrix, elements 186
Matrix, equality of two 189
Matrix, hat 220—221
Matrix, idempotent 221
Matrix, identity 197
Matrix, inverse 200—204
Matrix, multiplication by matrix 192—196
Matrix, multiplication by scalar 192
Matrix, nonsingular 201
Matrix, random 205—208
Matrix, rank 200
Matrix, scalar 197—198
Matrix, singular 201
Matrix, square 187
Matrix, subtraction 190—191
Matrix, symmetric 196
Matrix, theorems 204—205
Matrix, transpose 188—189
Matrix, vector 187—188
Matrix, zero vector 198—199
Maximum Likelihood Estimation 9—10
Maximum likelihood estimation of regression parameters 50—51
Mean of population, estimation of difference between two 14—16
Mean of population, estimation of single 11
Mean of population, test concerning, difference between two 14—16
Mean of population, test concerning, single 11—12
Mean response 41
Mean response, multiple regression, estimation 244
Mean response, multiple regression, joint estimation 245
Mean response, simple linear regression, interval estimation 75—76 217
Mean response, simple linear regression, joint estimation 157—159
Mean response, simple linear regression, point estimation 41—43
Mean square 46 88
Mean square, expected value of 90—91
Mean squared error of regression coefficient 395
Mean squared error, total of n fitted values 426
Measurement errors in observations 164—167
Method of steepest descent 479
Minimum absolute deviations method 411
Minimum sum of absolute deviations method 411
Minimum variance estimator 9
Minimum- -norm method 411
Multicollinearity 271—278 382—390
Multicollinearity, detection of 390—393
Multicollinearity, remedial measures 393—400
Multiple correlation see "Coefficient of multiple correlation"
Multiple regression see "Mean response" "Prediction "Regression "Regression "Regression "Selection
Multiplication theorem 3
Multivariate normal distribution 505
Noncentrality parameter 71
Nonexperimental data 35
Nonlinear regression model 468—469
Nonlinear regression model, inferences about parameters 480—483
Nonlinear regression model, least squares estimation 470—480
Nonsingular matrix 201
Normal equations 38
Normal error regression model see "Regression model"
Normal probability distribution 6—7
Normal probability distribution, table of areas and percentiles 517
Normal probability plot 118—120
Numerator degrees of freedom 8
Observation 25
Observational data 35
Observed value 41
Orthogonal polynomials 319
Outlier 114—116 123
Outlier, identification of 400—407
Overall F test 281 289
p-value 12—13
Paired observations 15—16
Partial correlation see "Coefficient of partial correlation"
Partial F test 281 289
Partial regression coefficient 229
Piecewise linear regression 346—350
Point estimator 38
Polynomial regression model 300—305
Power of tests for regression coefficients 71—72
Prediction bias 437
Prediction interval 77—78
Prediction of new observation, inverse 172—174
Prediction of new observation, multiple regression 246—247
Prediction of new observation, simple linear regression 76—82 159—160 218—219
Predictor variable 25 28
Probit transformation 366
Product operator 2
Pure error mean square 127—128
Pure error sum of squares 127
Quadratic effect coefficient 301
Quadratic form 215
Quadratic response function 301
Quadratic response function, estimation of maximum or minimum 317—319
Quantal response 354
random matrix 205—208
Random vector 205—208
Rank of matrix 200
Reduced model 95
Regression see "Mean response" "Prediction "Regression "Regression "Regression
Regression coefficients, multiple regression 227—229
Regression coefficients, multiple regression, danger in simultaneous tests 278—282
Regression coefficients, multiple regression, interval estimation 243
Regression coefficients, multiple regression, joint estimation 243
Regression coefficients, multiple regression, point estimation 238—239 263
Regression coefficients, multiple regression, tests concerning 243 285—286 289—293
Regression coefficients, multiple regression, variance-covariance matrix of 242 263
Regression coefficients, partial 229
Regression coefficients, simple linear regression 33—34
Regression coefficients, simple linear regression, interval estimation 65—67 69—70
Regression coefficients, simple linear regression, joint estimation 147—154 217
Regression coefficients, simple linear regression, point estimation 36—40 50—51 167—172 210—212 219—220
Regression coefficients, simple linear regression, tests concerning 67—68 71—72 92—94
Regression coefficients, simple linear regression, variance-covariance matrix of 216—217
Regression coefficients, standardized 261—263
Regression curve 27—28 see
Regression function 27—28
Regression function, comparison of two or more 343—45
Regression function, confidence band, simple linear regression 154—157
Regression function, confidence region, multiple regression 244
Regression function, estimated regression function 41—43
Regression function, test for fit 123—132 245—246
Regression function, test for regression relation 92—93 240—241
Regression function, transformations to linearize 134—141
Regression mean square 88
Regression model 26—29
Regression model, effect of measurement errors 164—167
Regression model, first-order autoregressive 448—450
Regression model, general linear 230—234 237—238
Regression model, multiple 226—230
Regression model, multiple in matrix terms 237—238
Regression model, multiple with interaction effects 232—237 335—339
Regression model, nonlinear 468—469
Regression model, polynomial 300—305
|
|
 |
Ðåêëàìà |
 |
|
|