|
 |
Авторизация |
|
 |
Поиск по указателям |
|
 |
|
 |
|
 |
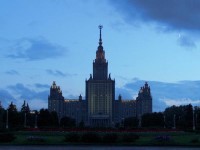 |
|
 |
|
Gruber M.H.J. — Regression Estimators: A Comparative Study |
|
 |
Предметный указатель |
Lagrange multiplier 6 74 77 85 90 129 237 238 289
Lauter, H. 20
Lauterbach, J. 15
Lawless, J.F. 24 26
Least favorable prior distribution 18
Least square estimator 4 69 70 82 303 305
Least square estimator for penalized splines 292 294—295
Least square estimator for the Kalman Filter 228—234
Least square estimator, ANOVA 253 256—257 267—268
Least square estimator, combined with other estimators 307—311 322 323
Least square estimator, comparison with Bayes estimator 118 122 153 169 171 174—179 193 201 203 214 220
Least square estimator, comparison with contraction estimator 141
Least square estimator, comparison with mixed estimator 104 105 155 161 171 188 189 201 307
Least square estimator, comparison with other estimators averaging over LINEX loss function 337 339 340—352
Least square estimator, comparison with other estimators averaging over Zellner's balanced loss function 316—317 319 324
Least square estimator, comparison with ridge estimator 129 237—239 273 341 342 383 384
Least square estimator, comparison with the Liu estimator 112
Least square estimator, derivation of 4—5
Least square estimator, distance from other estimators 374 383 384—387 389
Least square estimator, examples 9 72 79 124 157 298
Least square estimator, properties of 70—76 92 111 130 146 165
Lee, J. 31
Levy, M. 27 325
Lewis, F.L. 224 245
Lindley, D.V. 13 279
Linear admissible 17 144—146
linear combination 74 119 157 261 269 278 303 358
Linear estimator, admissible 12 21
Linear estimator, Bayes 12 18 24 69 70 91 93 145 153 154 165 208
Linear estimator, Bayes and empirical Bayes 233 234 239 248 263—267 273—278
Linear estimator, Bayes and penalized splines 291 292 296
Linear estimator, Bayes and the Rao distance 369
Linear estimator, Bayes for the LINEX loss function 338—340
Linear estimator, Bayes for Zellner's balanced loss function 304 307 315 324 338—340 369
Linear estimator, heterogeneous 14 17
Linear estimator, minimax 24 30 69 85 105 147
Linear restriction 16 18
Linnik, Y.V. 91
Lipovetsky, S. 29
Liski, E.P. 17
Liu, D. 31
Liu, K. 19 22 23 28 29 30 31 111 112 115 116 130 146 148 165 170 171 180 181 199
Loss functions, balanced 4 19 23 25 31—33 49 135 136 141 142 146 303—324
Loss functions, LINEX 4 15 20 22 23 26 27 32 49 135 136 143—144 146
Loss functions, matrix 58 59 142—143 303 318—320
Loss functions, predictive 14 111 141—142 196 198 303 304 318 320—324
Loss functions, quadratic 13 30 32 57—59 70 111 135—138 167 192 196—201 327 336
Loss functions, squared error 19 22 27 49 53 143—145 303 325 326 333 335 337—339 342 343 345
Lowerre, J.M. 11
Lu, Y. 31
Lynn, M.J. 24
Markiewicz, A. 21
Marquardt, D.W. 11
Maruyama, Y. 27
Mason, R.L. 20
Mathematical formulation of the Kalman Filter 225—228
Matrix loss function 58 59 142—143 303 318—320
Maximum likelihood estimator 10 26 34 54
Maximum likelihood estimator, inadmissibility of 53—56
Mayer, L.W. 11 23 28 29 110 165 207 310 376 377 382 383
McCabe, D.S. 283 284
McCulloch, C.E. 24
McDonald, G.C. 13 22 28
McKean, J.W. 143
Measures of efficiency of estimators 32 33 135—146 147
Measures of efficiency, average bias 150
Measures of efficiency, average MSE 13 32 50 52 136 138
Measures of efficiency, average MSE of Bayes estimator 153—155
Measures of efficiency, average MSE of contraction estimator 162—164
Measures of efficiency, average MSE of empirical Bayes estimator 265 276 278—282
Measures of efficiency, average MSE of Kalman Filter 239—242
Measures of efficiency, average MSE of Liu estimator 165
Measures of efficiency, average variance 147 151—152
Measures of efficiency, bias 9 13 17 18 20 27—30 52 111 135 137—138 148 168—170 196—198 336 337
Measures of efficiency, conditional MSE 32 135—137 167—201 202 209
Measures of efficiency, conditional MSE of Bayes estimator 52 139 214 215 218
Measures of efficiency, conditional MSE of least square estimator 148 218
Measures of efficiency, conditional MSE of ridge-type estimator 60 139
Measures of efficiency, conditional variance 137 148 149 168 195 197
Measures of efficiency, LINEX risk 326 331 334
Measures of efficiency, MSE averaging over a quadratic loss function 135 137 186
Measures of efficiency, squared bias 135 137 138 168—170 196 316
Measures of efficiency, variance of posterior distribution 50—52 57 138 151 158 333 336
Measures of efficiency, variance of prior distribution 151 369 372
Measures of efficiency, weighted bias 18 197 198
Measures of efficiency, weighted conditional variance 197
Measures of efficiency, weighted squared bias 196
Mehta, J.S. 14
Meinhold, N.D. 223 244
Mickens, R.E. 249
Minimax estimator 3 10 12—16 32 35 50 69
Minimax estimator and ridge-type estimators 3 109—116
Minimax estimator and the BLUR 296
Minimax estimator and the Kalman Filter 235—237
Minimax estimator and Zellner's balanced loss function 309—310
Minimax estimator, comparison with other estimators 93 94 105
Minimax estimator, efficiency of 13 47 165 208—210 220 224
Minimum variance unbiased linear estimator (MVUE) 74 89 116 117 130
Mitchell, A.F.S. 19 366 367
Mitra, S.K. 48
Mixed estimators 3 10 13 14 17 18 22 25 26 28 29 224 304 336 352 355
Mixed estimators, comparison with Bayes estimator 103—109
Mixed estimators, comparison with BLUR 295—297
Mixed estimators, comparison with least square estimator 228—234
Mixed estimators, derivation of 79—81
Mixed estimators, distance between 384—387 389
Mixed estimators, examples of 82—85 106—109 172—174 212—213
Mixed estimators, MSE of 155—157 167 171—174 187—192 201 203 210—213
Mixed estimators, variance of 147 160—161
Mixed model 18 271 272 291
Mixture of Bayes estimators 217—220
Mom, A. 30
Montano, R. 19
Montgomery, D.C. 22 23 254 259 271
Moore — Penrose inverse 11 42—48 98 107 131 192 256 257 297 305 310
Moore, G.P. 283 284
Morris, K. 253 267
MSE (mean square error) for incorrect prior assumptions 202—220
MSE (mean square error) of mixed estimator neglecting additional assumptions 187—192
MSE (mean square error) of parametric functions 12 13 194
MSE (mean square error), average MSE 136 138 147—149 205
MSE (mean square error), average MSE and average variance 151—152
MSE (mean square error), average MSE averaging over the LINEX loss function 336—340
MSE (mean square error), average MSE averaging over Zellner's balanced loss function 312—315
MSE (mean square error), average MSE compared to conditional MSE 179—181 215
MSE (mean square error), average MSE of BE 32 50 52 138 153—155
MSE (mean square error), average MSE of different estimators compared 13 157—165 215
MSE (mean square error), average MSE of EBE 265 276 278—282
MSE (mean square error), average MSE of Kalman Filter 239—242
MSE (mean square error), conditional MSE 135—139 148 167—201 209
MSE (mean square error), conditional MSE and average MSE compared 179—180
MSE (mean square error), conditional MSE and mixtures of BE 214 215 218
MSE (mean square error), conditional MSE averaging over quadratic loss functions 196—201
MSE (mean square error), conditional MSE of BE and LS compared 60 61 174—179 181—187
MSE (mean square error), predictive 17 198 200
MSE averaging over quadratic loss functions, individual 11 59 198 200 317
MSE averaging over quadratic loss functions, predictive 17 198 200 201
MSE averaging over quadratic loss functions, total 9 24 135 196 198 199 201
MSE neglecting prior assumptions 32 56 167—201
MSE, comparisons of BE (average) 32 66 157—162
MSE, comparisons of BE (conditional) 174 175—187 192—194
MSE, comparisons of mixed estimators (variance) 187—192
MSE, comparisons of ridge-type estimators and LS 12 13 15 162—165 167
MSE, expressions for Bayes estimator 153—155 168—171
MSE, expressions for mixed estimator 155—157 171—174
Multicollinear data 3 5 6 10 13 14 16 17 19 28 30 355 383
Multicollinear data, exact 5
Multicollinear data, near 5
Munoz, M. 19
Nagaoka, H. 19 355
| Namba, A. 23
Nishimura, T. 224
Nomura, M. 17
Non-estimable parametric function 71 149
Non-full rank, model 11—15 20—22 30 34 69 74 79 89 94 95 109 130 224 229 232—234 256
Non-full rank, prior dispersion 90 98—100 109—111 130 232—234
Non-full rank, prior ellipsoid matrix 26 86
Non-negative-definite matrix 35—38 56 58 59 137 164 175 188 206 289 294
Nonsingular matrix 41 43 45 103 210
Normal equation 5 7 34 72 73 79 254 260 267 273
Normal, population 19 26 50 56 326 328 331—334 336 337
Normal, prior distribution 31 50 245 371 374 375
Normal, random variable 259
Nyquist, H. 19 171
Obenchain, R.L. 13 15
Observation equation 244
Oh, H.S. 31
Ohtani, K. 20 344 347 352
Olman, V. 20 22
One-way ANOVA model 252 256 257—263
One-way classification 252 256 257—263
Optimization problem 77—79 85 89 90 93 96 116 168 217 220 232 237 257
Optimum scalar k or diagonal matrix K for LINEX loss function 343—344
Optimum scalar k or diagonal matrix K, squared error loss function 342—343
Optimum scalar k or diagonal matrix K, Zellner's balanced loss function 317—318
Ordering of matrices 35—38
Ordinary ridge regression estimator 21 28 69 82 110 111 138 139 148 165 170 178 180 183 216 250 298 371 383
Orthogonal contrast 254
Orthogonal matrix 45 71 82 111 121 124 190 192 311
Output equation 246
Owen, A. 29
Ozkale, S. 29 30 111
Palm, R. 20
Parker, D.F. 21 30 110 116
Patterned matrices 35 64—65 253 274
Peele, L. 13
Penalized spline 4 14 24 31 33 289—290 294—295 299
Pfeffermann, D. 11
Pliskin, J.L. 15
Pordzik, P.R. 18
Positive-definite matrix 36 60 95 99 210 235 248 251 296 340 360
Positive-semidefinite matrix 35—38 60—63 65 77 95 117 130 131 182 185 187 196 308
Posterior distribution, computation of 49—53 335
Posterior distribution, distance between 354 355 369—389
Posterior distribution, mean of 34 279 333 336
Posterior distribution, variance of 57 138 151 158 333 336
Posterior mean 334 336
Posterior variance 152 336
Prediction error 12
Pretest estimator 25 219 266
Price, M.J. 13
Principal minor 35 36
Prior, assumptions 56 136—141 148 165—167 171—174 188—192 201—209 224 226 242—244 263 273 306
Prior, dispersion 14 101—103 117—120 157—159 165 174 179 185 193—195 197 248 310 369
Prior, distribution 13 21 31 49 52 91 116 126 147 151 202 214 333 354
Prior, information 3 4 12 20 21 34 51 94 136 156 167 168 197 239 253 263
Prior, mean 15 70 88 98 105 116 120 139 155 157 158 167 174 214 314 336 370—373
Prior, parameters 31 85 147 157 177 253
Prior, variance 151 369 372
Quadratic form 20 22 35 36 213 270
Quenouille, M. 16 18
Ramanathan, T.V. 30
Random sample 49 50 52 56 75 152 328 335
Random-effects model 262 271 297
Randomized block design 252 253 267 270
Rao, C.R. 48 60 64 70 76 89 91 94 95 96 109 157 178 180 218 295 354
Rao, J.N.K. 24
Rao, P S.R.S. 24
Rayner, R.K. 19
Recursive Bayes estimation 224
Recursive least square estimator 228—234
Recursive ridge regression 248—251
Reflected loss function 332
Relative savings loss 253 266
Ren, C. 26
Reparameterization of a non-full-rank model to one of full-rank 121 123 125 127 131 185
Ridge regression estimators 4 7 12 13 15 17—20 22—24 27—30 95 106 110 178
Ridge-type estimators and LINEX loss 342—352
Ridge-type estimators and penalized splines 283—299
Ridge-type estimators as special cases of more general classes of estimators 94 96 98 109—116 248—251
Ridge-type estimators, admissible 53 134 144—146
Ridge-type estimators, comparison of efficiency 60 135 162—163 165 176—179 181—182 194—196 207—208 342—352
Ridge-type estimators, contraction estimators 110 154 169 207—208
Ridge-type estimators, distance between 33 376—379 382—384
Ridge-type estimators, generalized contraction estimators 111 138—339
Ridge-type estimators, generalized ridge estimators 109 129—130
Ridge-type estimators, historical prospective 11 12 13 17 21
Ridge-type estimators, Liu (two parameter) 111—112 165 170
Ridge-type estimators, need for the study of 4—10
Riquan, Z. 31
Robinson, G.K. 18 24
Robustness of an estimator with respect to prior information 14 15 32 202 204
Robustness of assumptions 224 242—244
Robustness of prior information 16 32
Robustness of the Kalman Filter with respect to initial prior assumptions 224 242—244
Ruppert, D.W. 283 289 298
Ryan, T.P. 13
Sakar, N. 21
Saleh, A.K.M.E. 26 219 266
Sample information 82 83
Santos, S. 19
Saxena, A. 14
Schaffrin, B. 25 31
Schipp, B.G. 16
Searle, S.R. 24 71
Shao, P.Y.S. 55
Shatzoff, M. 9
Shrinkage estimators 4 11 12 22 27 29 377
Shukur, G. 27
Signal-to-noise ratio 30 170 196—197
Significance tests 253 266 288
Silvapulle, M.J. 18
Silvey, S.D. 10
Simulation studies 9 12 17 30 31
Singh, B. 17 30
Singpurwalla, R.J. 223 244
Singular value decomposition (SVD) and Bayes, mixed, and ridge-type estimators 113—114 123 125—128 158—159 311
Singular value decomposition (SVD) and estimable parametric functions 72 255 256
Singular value decomposition (SVD) for generalized inverses 34 43—48
Singular value decomposition (SVD) for the exchange of sample and prior in formation 106—108 191
Singular value decomposition (SVD) in distance functions 367 368
Singular value decomposition (SVD) in establishing important results 58 62 97 104 121 218
Singular value decomposition (SVD), computation of 38—41
Smith, A.F.M. 11 13
Soong, T.T. 224
Sorenson, H.W. 223
Space 41 278 357—359
Speed, E.M. 24
Splines 4 14 24 25 27 31 33 283—299
Springer, E.A. 27 325 332
Srivastava, M.S. 26 27
Stahlecker, P. 15 16 22
Stapasson, J. 19
Starke, H.R. 31
Stein estimators 10 12 20 23 27 53—55 94 110 140 219 251 259 265 277 278
Stein, C. 10 12 13 15 20 22 23 25 27 34 54 55 94 110 111 140 219 251 259 265 275 277 278 322
Steinour, H.H. 31
Stevens, P.J. 223
Stochastic prior constraints 22 121 211
Stochastic prior information 82—84 103—106 137 210
Strawderman, W.E. 27 55
Styan, G. 28
subspace 41 126 127 128 131
Sufficiency principle 279
Sufficient statistic 21 279
Sum of squares 29 75 84
Sum of squares, between 258
Sum of squares, block 270
Sum of squares, error 253 258 264 270 290 304
Sum of squares, regression 258
|
|
 |
Реклама |
 |
|
|