|
 |
Àâòîðèçàöèÿ |
|
 |
Ïîèñê ïî óêàçàòåëÿì |
|
 |
|
 |
|
 |
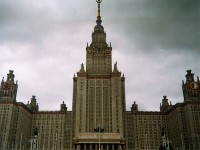 |
|
 |
|
Wallace C.S. — Statistical and Inductive Inference by Minimum Message Length |
|
 |
Ïðåäìåòíûé óêàçàòåëü |
AC see “Algorithmic complexity”
Afterlife theory 397
AIC (Akaike information criterion) 303
Akaike information criterion (AIC) 35 303
Akaike, H. 35
Algorithmic complexity (AC) 100—110 311
Algorithmic complexity (AC), explanations and 118—121
Algorithmic complexity (AC), Shannon information versus 107—110
Algorithmic Probability (ALP) 406
Allison, L. 209 274
ALP (Algorithmic Probability) 406
Alphabet 58
Alternative priors 350 353
Ancillary statistic 242
Approximate theories 14
Arcs 305—306
Arithmetic coding 73—76
Arrow of Time, Thermodynamic 337—384
assertion 152 see first
Assertion code for probabilistic finite-state machines 308—309
Assertion, imprecise, of discrete parameters 284—286
Assertion, length of 235
Asymmetry, reasons for 367—369
Atom count difference 383
Bakus — Naur form (BNF) 306—307
Barron, A.R. 311
Baxter. R.A. 274
Bayes Information Criterion (BIC) 303
Bayes posterior density 182
Bayes' theorem 22
Bayesian decision theory 40—45
Bayesian inference 1 35—40
Bayesian inference, relation to 116—118
Bernardo, J.M. 47
Bernoulli sequence 147
Best explanation of data 1
Beta density 246
beta function 246
Beta prior density 47
Bhansali, R.J. 35
Bibby, J.M. 300
BIC (Bayes Information Criterion) 303
Binary codes 59—63
binary digits 59
Binary sequence segmentation problem 321—326
Binary tree codes 95—96
Binomial distributions 24
Binomial distributions, irregularities in 248
Binomial distributions, negative 253
Binomial example 157—160
Binomial example, using sufficient statistics 163—164
Bit 66
Blurred images 208—209
BNF (Bakus — Naur form) 306—307
Boltzmaiiii, Stefan 338—339
Boltzmann's constant 88
Boulton, D.M. 81 401
Boundary rule, for growing data groups 171—173
Boundary rule, for ideal data groups 198—199
Carnap, R. 5
Categories 315
Categories, regression in 320
Cauchy density 32
Causal explanations 365—367
Causal nets 326—336
Cause, effect and 365—367
Chaitin, G.J. 3—4 57 102 109 275 401
Chickering, D.M. 330
ChiSquared form 34
Class distributions at leaves, coding 317 318
Class labels 276
Class labels, Fisher Information with 291 293
Class labels, surrogate estimate 288—289
Class proportions 294
Classification trees and nets 314 320
Classified models, summary of 293 294
Classified models, unclassified models versus 295 297
Cleary. J.G. 73
Clocks, example of 353—355
Closed systems 339 340
Coarse data, normal distribution with 265—266
Code length of imprecise discrete estimates 286—288
Code tree 61
Code word in Huffman code 282
Codeable estimates 213—215
codes 59
Codes, binary tree 95—96
Codes, feasible, for infinite sets 96 98
Codes, for infinite sets 91—92
Codes, non-biuar.y 77—78
Codes, optimal see “Optimal codes”
Codes, universal 98 100
Codes, universal, in theory descriptions 115—116
Coding probability 149—150
Coding probability, prior probability density and 222
Coding scheme. MML 222—228
Coding transitions 312—313
Coding trick 281—284 293
Coding, arithmetic 73 76
Coding, class distributions at leaves 317—318
Coding, multi-word messages 72 73
Coding, of data 146—147
Coding, of inferences 148—150
Coding, random, of estimates 210
Coding, tree structure 316—317
Collision table 370 375
Communication of information 57—58
Complexity Approximation Principle 405
Computer process, past of a 375
Concatenation 119
Concentration parameter 259
Conditional probability 21
Confidence 30
Confidence interval 30
Conjugate priors 46—48
Conjugate priors, for multivariate Normal distribution 261—264
Conjugate priors, for Normal distribution 258—264
Conjugate priors, invariant 52—53 261
Consequential propositions 7
Continuous data, SMML explanation for 166—187
Continuous distributions 24—25
Continuous random variables 20
Conway, J.H. 178 257
Cost function 54
Counter-instances 10
Cover, T.M. 98 311
Cross-Validation (CV) criterion 321 323
Curved-prior message length, MML 236—237
Cut points 321—322
CV (Cross-Validation) criterion 321—322
DAG (directed acyclic graph) 326—327
Data acquisition 397
Data groups 222
Data groups, growing, boundary rule for 171—173
Data groups, ideal 198—199
Data representation invariance 187—188
Data, coarse, normal distribution with 265—266
Data, coding of 146—147
Data, continuous, SMML explanation for 166—187
Data, discrete, SMML explanation for 153—166
Data, explanation of 359
Data, perturbed, normal distribution with 264—265
Data, possible, set X of 144—145
Data, probabilistic model of 146
decimal numbers 59
Decision graphs 318—320
Decision tree explanation 315—316
Decision trees 315
Deduction, of past disorder 345—355
Deduction, uses of 361—302
| Deduction, with deterministic laws 346—348
Deduction, with non-deterministic laws 348—350
Deductive reasoning 5
Defining propositions 7
density 25
Descriptive MML theory 385—399
detail 152 see second
Detail length 235 325—326
Deterministic laws 343—344
Deterministic laws, deduction with 346—348
Deterministic laws, induction of the past with 363—365
Devolution 346
diatomic molecules 374—375
Dirac delta function 193
Directed Acyclic Graph (DAG) 326—327
Disc collision model 351
Discrete data, SMML explanation for 153—166
Discrete distributions 23—24
Discrete estimates, imprecise, code length of 286—288
Discrete hypothesis sets 156
Discrete parameters, imprecise assertion of 284—286
Discrete random variables 20
Disorder 337
Disorder, entropy as measure of 341—343
Disorder, past, deduction of 345—355
Dissipation 361
Dissipative laws 345
Distributions 23
Distributions, binomial see “Binomial distributions”
Distributions, distributions information content of 81—87
Distributions, entropy of 89
Distributions, infinite entropy 94
Distributions, multinomial see “Multinomial”
Distributions, Normal see “Normal distributions”
Distributions, predictive 206
Distributions, probability see “Probability distributions”
Distributions, uniform, of known range, mean of 183—187
Dowc, D.L. 203 209 216 219 252 268—269 274 323—326
Dowe's approximation to message length 209—213
Dowe's construction, uncertainty regions via 216
Downham, D.Y. 35
Educated Turing machines (ETM) 130—131
Effect, cause and 365—367
EM (Expectation Maximization) algorithm 276 279
Empirical Fisher Information 240—245
Empirical Fisher Information, transformation of 243—244
Empirical information matrix 241
entropy 51 87—91 337
Entropy, as measure of disorder 341—343
Entropy, increasing 343—344 376 384
Entropy, of distributions 89
Entropy, time reversal and 350
Equilibrium 341—342
Equivalence sets 329
Equivalence, partial order 330
Equivalence, structural 330 331
escape sequence 136
Estimate spacing, precision of 238—240
Estimate(s) 30
Estimate(s), class label, surrogate 288—289
Estimate(s), codeable 213—215
Estimate(s), imprecise discrete, code length of 286—288
Estimate(s), random coding of 210
Estimate(s), Schou 268
Estimation of Normal mean with Normal prior 173—177
Estimator 31
Estimator function 154
ETM (Educated Turing Machines) 130—131
Euclidean distance 178
Euler's constant 179
Evolution probability 348
Evolutionary induction 396—397
Exceptions 18
Expectation 25—27
Expectation Maximization (EM) algorithm 276—279
Expected string length 64
Expected value of loss function 189
experiments 397—399
Explanation length 143 160 331
Explanation message 16—19
Explanation message, shortest 143
Explanation structure, three-part 242
Explanation(s) 14—19
Explanation(s), algorithmic complexity and 118—121
Explanation(s), first part of 112—114 121—123
Explanation(s), of data 359
Explanation(s), second part of 110—112 120—121
Explanatory power 13 16
Factor analysis model 297—303
Factor analysis model, defining equations for 299
Factor analysis model, MML 300—303
Factor loads 298
Factor scores 298
Fair's algorithm 164—165
Fair, G.E. 159 164—165 172
Falsifiable propositions 10—11
Falsifying data 12
Familv of models 28
Fano, R.M. 70—72
Feasible codes for infinite sets 96 98
Fermi — Dirac statistics 378
Finite-State Machines (FSMs) 127—128 305—314
Finite-state machines (FSMs), alternative expression for 228—229
Finite-state machines (FSMs), empirical see “Empirical Fisher Information”
Finite-state machines (FSMs), Fisher determinant 227
Finite-state machines (FSMs), Fisher Information 48 225 411—412
Finite-state machines (FSMs), for mixtures 290—291
Finite-state machines (FSMs), less-rednndant code for 309—310
Finite-state machines (FSMs), safer empirical approximation to 244—245
Finite-state machines (FSMs), transparency and redundancy in 310 312
Finite-state machines (FSMs), with class labels 291—293
Fisher matrix 232
Fitzgibbon, h. 209 274
Fnput tape, Turing machines 101
Formula I1A 226
Formula I1A, for many parameters 210—241
Formula I1B 226
Formula I1C 243
Freeman, P.R. 226—227 300
FSMs see “Finite-state machines”
Function approximation 272—275
future 368
Gaines, B.R. 313
Gammerman, A. 405
Gas simulations 370—375
Geometric constants 257—258
Glymour, C 327
God theory 397
Grammars, regular see “Regular grammars”
Griinwald, P.D. 227
Group 50
Growing data groups, boundary rule for 171—173
Hexagonal Voronoi regions 181
Huffman code 70—71
Huffman code, code word in 282
Huffman. D.A. 70—73 78 103 107 282 284—285
Human induction 394 396
Hutter, M. 403—405
Hypothesis space, partitions of 213—215
Hypothesis space, uncertainty regions in 214—215
Ideal data groups 198—199
Ideal group (IG) estimator 197—200
Ideal group (IG) estimator for Neyman — Scott problem 201—202
IG see “Ideal group estimator”
Images, blurred 208—209
Imprecise assertion of discrete parameters 284—286
Imprecise discrete estimates, code length of 286—288
Independence 22
Induction 1
Induction, evolutionary 396 397
|
|
 |
Ðåêëàìà |
 |
|
|