|
 |
Àâòîðèçàöèÿ |
|
 |
Ïîèñê ïî óêàçàòåëÿì |
|
 |
|
 |
|
 |
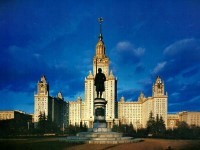 |
|
 |
|
Little R.J.A., Rubin D.B. — Statistical analysis with missing data |
|
 |
Ïðåäìåòíûé óêàçàòåëü |
Acceleration techniques 27
Additive model 253
Adjusting standard errors for filled-in missing values 32—34
Adjusting sums of squares for filled-in missing values 34—36
Adjustment cell 56—57 254
Adjustment cell, models 250—253 260—261
Afifi, A. A. 6 18 39 48
Aitkin, M. 208 216
Algorithms, iterative 128—129 (see also EM algorithm Newton-Raphson Scoring
Allan, F. G. 26 36 37
allocation (see Filling in for missing values)
ALLVALUE estimates 42
Amemiya, T. 223 224 241
Analysis of covariance (Ancova) 27—30
Analysis of variance (Anova) 14 21—38 94 152—153 203—206
Analysis of variance (ANOVA), mixed effects 149—152
Analysis of variance (ANOVA), random effects 149—152
Anderson, R. L. 22 36
Anderson, T. W. 82 95 98 119 120 124 125 204 216
Approximate Bayesian bootstrap 258—259
Approximate covariance matrix 98 154
AR1 Model 163
Association, in contingency tables 185
Asymptotic approximations, accuracy 105—107
Asymptotic covariance matrix of parameters or estimates 84—86
Asymptotic covariance matrix of parameters or estimates for categorical data 180
Asymptotic covariance matrix of parameters or estimates for general missing data pattern 137 139
Asymptotic covariance matrix of parameters or estimates for multiple regression 154
Asymptotic covariance matrix of parameters or estimates for multivariate normal data 145
Asymptotic normality 84—86
Asymptotic standard errors (see Asymptotic covariance matrix of parameters or estimates)
Augmented covariance matrix, definition 114
Autoregressive model 158 161 165
Autoregressive model, moving average (ARMA) models 162
Available-case methods 41 43 49 109—112 169
Azen, S. 43 48 49
Baghelai, C. 169
Bailar, B. A. 65 71
Bailar, J. C. 65 71
Bailey, L. 65 71
Baker, S. 237 240 241
Balanced repeated replication 71
Banded covariance structure 158 161
Bard, Y. 93 95
Bargmann, R. W. 145 169
Bartlett, M. S. 27 29 36 37
Bartlett’s method 27—30
Baum, L. E. 129 139
Bayesian bootstrap 265
Bayesian inference 53 84—88 93 104—107 230—235 244—265
Beale, E. M. L. 129 139 144 145 154 155 168 170
Beaton, A. E. 112 124
Bemdt, E. B. 128 139 224 241
Bent, D. H. 19
Bentler, P. M. 168 170
Beta distribution 95
Between-imputation variance 257
Bias due to nonresponse 15—17 53 Nonignorable
Bias vs. variance 70
Binomial distribution 140
Bishop, Y. M. M. 172 173 186 187 193
Bivariate data 13—16 49
Bivariate data, normal data 14 83 92 96 125—126 169—170
Bivariate data, normal data, EM algorithm 132—134
Bivariate data, normal data, ML estimation 98—102
Bivariate data, normal data, precision of estimation 102—107
Bivariate data, normal monotone data 98—107 115
Bivariate data, normal stochastic censoring model 224
Bivariate data, time series 167
BMDP statistical software, 4F 189
BMDP statistical software, 8D 41 42 126
BMDP statistical software, AM 4
Bobula, J. 169
Bootstrap 71 265
Box — Cox power transformation 228—229
Box — Jenkins models 162
Box, G. E. P. 87 95 104 124 125 162 168 227 241 264 265
Box, M. J. 93 95
Brown, D. T. 204 216
Brownlee, K. A. 152 168
Buck, S. F. 44 45 46 47 48 49
Buck’s method 45 47 49
Caines, P. E. 165 168
Calibration experiment 14
Candidates for imputation 66
Canonical correlation 142
Cassell, C. M. 58 71 73
categorical data 4—5 95 131—132 171-194 196—217 261
Categorical data, independence model 182 253
Categorical data, nonignorable models 235—241 (see also Loglinear models)
Cauchy distribution 95
Censored data 9—13 91—92 141 219 221—230
Censored data with known censoring points 10 94—95 141 222—223
Censored data with stochastic censoring points 10—12 223 224—225 230
Censored data, exponential sample 94—95 141 222
Central limit theorem 52 85
Chen, T. 183 193
Chi-squared statistics (see Likelihood ratio statistic Goodness-of-fit
Circular symmetry pattern 147
Clarke, W. R. 4 5 19
Cluster analysis with missing data (see Mixture models)
Cluster samples 69
Cochran, W. G. 21 31 32 36 37 51 53 63 66 71 101 124 125 150 169 247 254 264
Cold deck imputation 60
Colledge, M. J. 66 71
Comparison of missing-data methods 109—112 228 262—264
Compensatory reading example 234—235
Complete-case methods 40 41 49 110—112
Complete-data likelihood 130 134
Complete-data sufficient statistics 138
Compound symmetry 158
Computational strategies 127—141
Computer packages, missing-data methods in 3—4 6
Conditional independence 148 239
Confirmatory factor analysis 149
Consistent estimates from incomplete data (see Inference based on maximum likelihood theory Missing
Contaminated normal model 209—216
Contaminated normal model, multivariate normal model 211—216
Contingency table (see Categorical data Loglinear
Contrasts 32—36
Convergence, quadratic 140
Corby, C. 65 71
Correlations, estimates from incomplete data 40—41 42 43 46 105—107
Correlations, inestimable 120—124
Cosier, J. 169
Counted data (see Categorical data)
Covariance components models 150
Covariance matrix of estimates 84—85
Covariance matrix, estimation from incomplete data 39—41 142—145
Covariance matrix, robust estimation 211—215 (see also Asymptotic covariance matrix of parameters or estimates)
Cox, D. R. 79 84 95 227 241
Cox, G. 21 31 32 36
Current Population Survey 65 227
Curry, J. 43 48 49
Darwin’s data 208
Data matrix 3 19
David, M. H. 60 61 62 71 229 241
Davies, O. L. 21 36
Dawid, A. P. 54 71
Day, N. E. 207 216
Degrees of freedom for lack of fit in contingency tables 186—188 192 237—241
Degrees of freedom, corrections 23 29 32 34 35 144
DeGroot, M. H. 93 95
Deleting observed values (see Discarding data)
Dempster, A. P. 6 18 43 48 112 124 129 130 131 135 136 137 139 145 150 152 168 209 210 216
Density function 79
Dependent variables, missing values in 21—38 152—157 196 203 220-230 232—235
Design weights 6
Design-based inference in surveys (see Randomization inference for surveys)
Dichotomous data 48 (see also Categorical data)
| Discarding data 6 19 109—112
Discrete data (see Categorical data)
Discriminant analysis 142 196—206
Distinct parameters 90 97
distribution 209
Dixon, W. J. 4 19 41 42 48 124 126 189 193
Donor for imputation 63 66
Double sampling 9 254
Draper, N. R 23 36 93 95 117 124
Dummy variable regression 108 254
E step (expectation step) 130 (see also EM algorithm)
EA’s (see Enumeration areas (EA’s))
editing (see Outliers)
Educational testing 121—124
Educational testing, examples 148
Efficiency 175
Elashoff, R. M. 6 18 39 48
EM algorithm 18 26 129—139 140
EM algorithm for exponential families 138—139
EM algorithm for nonignorable missing data 220—221
EM algorithm for specific missing-data problems factor analysis 148—149
EM algorithm for specific missing-data problems grouped exponential sample 221—222
EM algorithm for specific missing-data problems grouped normal data with covariates 222—224
EM algorithm for specific missing-data problems log-linear models 187—191
EM algorithm for specific missing-data problems logistic regression 196
EM algorithm for specific missing-data problems missing outcomes in ANOVA 152—153
EM algorithm for specific missing-data problems mixed continuous and categorical data 195—208
EM algorithm for specific missing-data problems multivariate non-normal data 209—216
EM algorithm for specific missing-data problems multivariate normal data 143—145
EM algorithm for specific missing-data problems multivariate regression 155—157 215—216
EM algorithm for specific missing-data problems partially classified contingency tables 181—185 240
EM algorithm for specific missing-data problems regression 153—154 196
EM algorithm for specific missing-data problems repeated measures models 158
EM algorithm for specific missing-data problems restricted covariance matrix 146—148 158
EM algorithm for specific missing-data problems selection models 224—225
EM algorithm for specific missing-data problems time series 163—168
EM algorithm for specific missing-data problems variance components 149—152 143—145
EM algorithm for specific missing-data problems with follow-up data 243
EM algorithm, convergence 129 130 135 137 140
EM algorithm, rate of convergence 137—138
EM algorithm, theory 134—137 138—139 220-221
Empirical Bayes model 149—152
Enumeration areas (EA’s) 68
Ernst, L. R. 60 71
Expectation-maximization algorithm (see EM algorithm)
Expected mean squares 152
experiments 16 21—38 152—153
Exploratory factor analysis 149
Exponential data 80 82 86 90—92
Exponential data with censored values 94—95 141 222
Exponential data with grouped values 221—222
Exponential distribution 221—222
Exponential family 136 138—139 140 197 204
F distribution 23
Factor analysis 148—149 158 170
Factor analysis with missing data 149
Factor analysis, loading matrix 148
Factor analysis, score matrix 148
Factored likelihood 15 97—98
Factored likelihood for bivariate normal data 98—101
Factored likelihood for mixed continuous and categorical data 206—207 217
Factored likelihood for monotone data 107—108 215—216
Factored likelihood for multivariate normal data 108—112
Factored likelihood for partially classified contingency tables 172—181 239
Factored likelihood for special nonmonotone patterns 119—124
Factored likelihood, computations via SWEEP 115—119 122—124
Factorization table 126
Fay, R. E. 237 241
Fienberg, S. E. 172 173 183 186 187 193
File matching 121
Filling in for missing values 6 21—38 43 47 60—67 254—259
Filling in for missing values and iterating 26—27 129
Filling in for missing values and using complete data methods 30—37
Filling in for missing values and using formulas 26
Filling in for missing values, conditional means, as from regression 25 45 47 61 102 253—255
Filling in for missing values, least squares estimates 25
Filling in for missing values, relationship with weighting 62
Filling in for missing values, stochastically, to preserve distributions 47 60 61 62—67 254—259
Filling in for missing values, unconditional means 44
Finite population 50
Finite population, correction 247
Finite population, inference model-based 244—265
Finite population, inference randomization-based 50 75
Fisher, R. A. 26
follow-ups 243 262—264
Ford, B. N. 60 71
Forecasting 165
Fraction of information missing 257
Frequency data (see Categorical data)
Frequentist inference 84—88
Fuchs, C. 175 178 189 192 193
Fully missing variables 146
Fully normal imputation 259 265
Galke, W. 223 241
Gamma distribution 140 210
Gaussian distribution (see Normal data)
GEM algorithm (see Generalized EM algorithm)
General location model 196 206 217
General missing-data patterns 17—18
General state space models 162
Generalized EM algorithm 135 158 204
Glynn, R. 262 264
Goel, K. 93 95
Goodman, C. 169
Goodman, L. A. 17 186
Goodness-of-fit statistics 186 192
Goodnight, J. H. 112 124
Goodrich, R. L. 165 168
Greenlees, W. S. 230 241
Grouped and rounded data 211 221 223
Grouped and rounded data, exponential sample 221—222
Grouped and rounded data, normal data 222 223
Growth curve models 158—161
Gupta, N. K. 165 168
Haberman, S. J. 186 193
Haitovsky, Y. 43 48 49
Hajek, J. 52 71
Hall, B. 139 241
Hall, R. 139 241
Hansen, M. H. 51 71 254 264
Hartley, H. O. 6 19 26 36 129 139 145 168 182 193
Harvey, A. C. 162 168
Hasselblad, V. 223 241
Hausman, J. A. 139 241
Healy, M. J. R. 26 36 153 168
Heckman, J. 224 229 230 241
Heckman’s two-step method 229—230
Helms, R. W. 157 168
Herson, J. 254 264
Herzog, T. N. 61 72 255 256 258 264
Heterogeneity of variance 254
Hierarchical loglinear models 186 235
Hinkley, D. V. 79 84 95
Historical heights 12—13
Hocking, R. R. 6 19 145 168 177 179 193
Holland, P. W. 148 168 172 173 186 187 193
Horvitz — Thompson estimator 55 56 69 73
Horvitz, D. G. 55 56 69 72 73
Hot deck imputation 6 60 62—67 75 256 258 265
Hot deck imputation within adjustment cells 65
Hot deck imputation, increase in variance of estimation 64—65 75
Hot deck imputation, metric, nearest neighbor 65—67
Hot deck imputation, random sampling with replacement 63—64
Hot deck imputation, random sampling without replacement 64—65
Hot deck imputation, sequential 65—67
Hull, C. H. 19
Hunter, W. G. 93 95
Hurwitz, W. N. 51 71
Hypothesis testing 87—88 (see also Likelihood ratio statistic)
Ignorable missing-data mechanism 9 10—13 55 248 250—255
|
|
 |
Ðåêëàìà |
 |
|
|