|
 |
Авторизация |
|
 |
Поиск по указателям |
|
 |
|
 |
|
 |
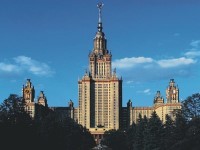 |
|
 |
|
Mitchell T.M. — Machine Learning |
|
 |
Предметный указатель |
TD-Gammon, and Backpropagation algorithm in 384
Temporal credit assignment in reinforcement learning 369
Temporal difference learning 383—384 386—387
Terms in logic 284 285
Text classification, naive Bayes classifier in 180—184
Theorem of total probability 159
Tournament selection 256
Training and validation set approach 69 See
Training derivatives 117—118
Training error in multilayer networks 98
Training error in multilayer networks, alternative error functions 117—118
Training error of continuous-valued hypotheses 89—90
Training error of discrete-valued hypotheses 205
Training examples 5—6 17 23 See
Training examples in PAC learning 205—207
Training examples in PAC learning, bounds on 226
Training examples, explanation in Prolog-EBG 314—318
Training examples, Voronoi diagram of 233
Training experience 5—6 17
Training values, rule for estimation of 10
True error 130—131 133 137 150 204—205
True error in version spaces 208—209
True error of two hypotheses, differences in 143—144
Two-point crossover operator 255 257—258
Two-sided bounds 141
Unbiased estimator 133 137
Unbiased learners 40—42
Unbiased learners, sample complexity of 212—213
Uniform crossover operator 255
Unifying substitution 285 296
Unsupervised learning 191
Utility analysis in explanation-based learning 327—328
Validation set See also "Training and validation set approach"
Validation set, cross-validation and 111—112
| Validation set, error over 110
Vapnik — Chervonenkis (VC) dimension See "VC dimension"
Variables in logic 284 285
Variance 133 136—138 143
VC dimension 214—217 226
VC dimension of neural networks 218—220
VC dimension, bound on sample complexity 217—218
VC dimension, definition of 215
Version space representation theorem 32
Version spaces 29—39 46 47 207—208
Version spaces, Bayes optimal classifier and 176
Version spaces, definition of 30
Version spaces, exhaustion of 208—210 226
Version spaces, representations of 30—32
Voronoi diagram 233
Weakest preimage 316 329
Weight decay 111 117
Weight sharing 118
Weight update rules 10—11
Weight update rules, Backpropagation weight update rule 101—103
Weight update rules, Backpropagation weight update rule in KBANN algorithm 343—344
Weight update rules, Backpropagation weight update rule, alternative error functions 117—118
Weight update rules, Backpropagation weight update rule, optimization methods 119
Weight update rules, Backpropagation weight update rule, output units 171
Weight update rules, delta rule 11 88—90 94
Weight update rules, gradient ascent 170—171
Weight update rules, gradient descent 91—92 95
Weight update rules, linear programming 95
Weight update rules, perceptron training rule 88—89
Weight update rules, stochastic gradient descent 93—94
Weighted voting 222 223 226
Weighted-Majority algorithm 222—226
Weighted-Majority algorithm, mistake-bound learning in 224—225
Widrow — Hoff rule See "Delta rule"
|
|
 |
Реклама |
 |
|
|